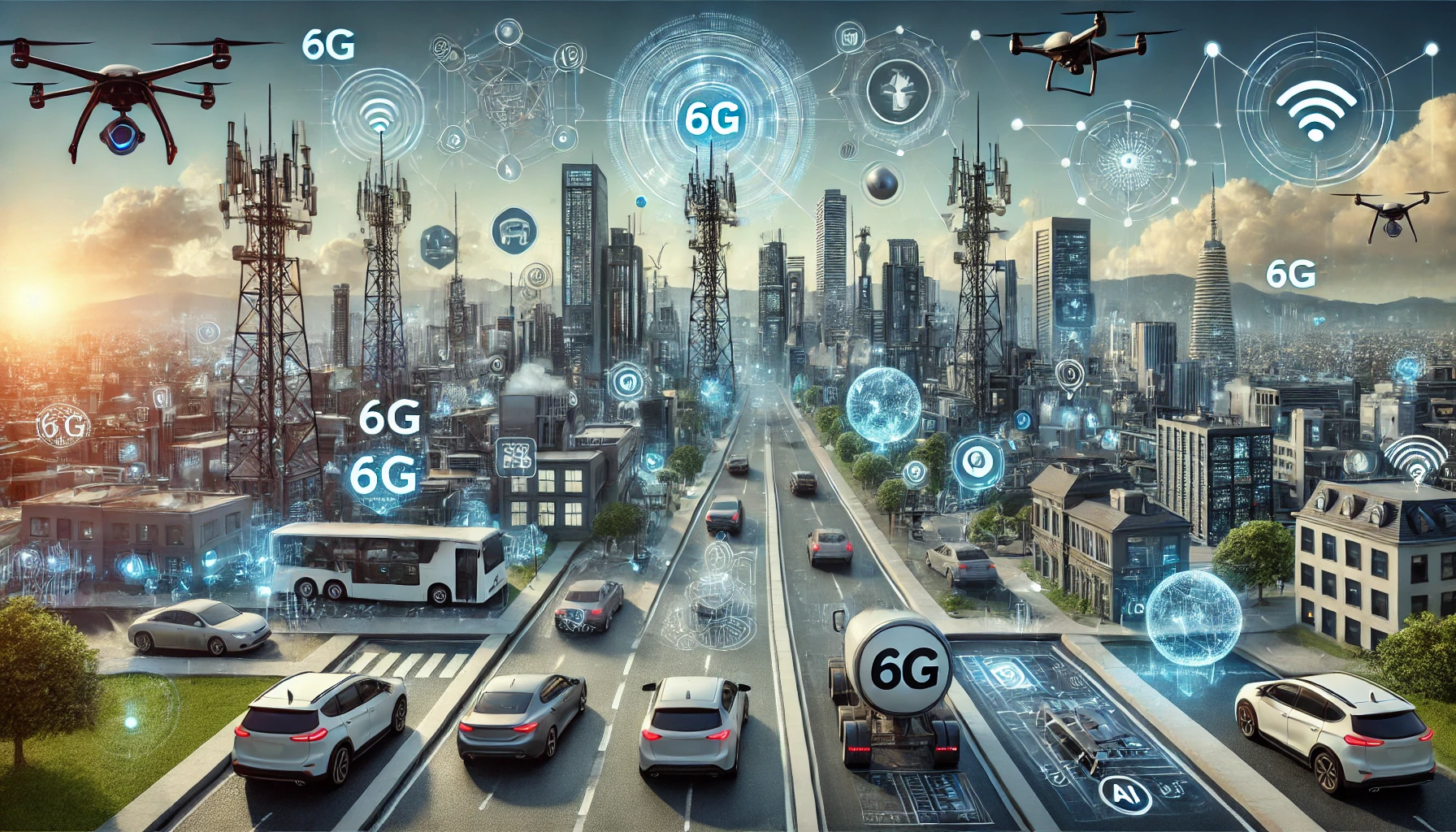
Astudy by several researchers from Seoul National University (SNU) and the Massachusetts Institute of Technology (MIT), discusses how integrating sensing technologies with computer vision (CV) can revolutionize 6G wireless communication systems. This combination, known as sensing and CV-aided wireless communications (SVWC), promises to offer substantial performance improvements over existing 5G technologies, addressing the increasing demand for high data rates driven by services like digital twins and the metaverse. These immersive mobile services require data rates far beyond what is currently available, necessitating the exploration of ultra-high frequency spectra, including millimeter-wave (mmWave) and terahertz (THz) bands. However, higher frequencies pose challenges such as reduced communication distances due to severe path loss and strong directivity.
Unleashing the Power of Sensing and Computer Vision
Sensing technology plays a crucial role in detecting and capturing visual, auditory, and tactile information about the physical world. Computer vision techniques then analyze this information to understand and interpret the environment. The CV process involves three main steps: vision acquisition, vision processing, and decision-making. Vision acquisition captures two-dimensional and three-dimensional sensing information using devices like RGB cameras, LiDAR, and infrared cameras. Vision processing extracts features from the sensing data using advanced deep learning (DL) models, such as convolutional neural networks (CNN) and Transformers, which identify patterns and objects in the data. Decision-making uses these extracted features to perform tasks like image classification, object detection, and semantic segmentation.
Transforming Wireless Communication with SVWC
SVWC leverages these capabilities to enhance wireless communication by providing fast and accurate identification of wireless environments and objects, as well as contextual understanding of their interactions. This integration can significantly improve various aspects of wireless communication. For instance, in beam management, which is essential for compensating path loss in mmWave and THz communications, SVWC enhances accuracy and reduces latency by directly detecting the position of mobile devices and generating directional beams toward them. This approach eliminates the need for time-consuming two-step beam management processes used in 5G.
Proactive Solutions for Seamless Connectivity
In cell association, SVWC predicts the line-of-sight (LoS) and non-line-of-sight (NLoS) status between base stations and mobile devices, enabling proactive management of cell association. This ensures seamless connectivity and improved link quality, preventing sudden link deterioration that can occur with conventional reactive methods. SVWC also aids in environment-aware channel estimation by using CV techniques like neural radiance fields (NeRF) to construct three-dimensional representations of wireless environments. This approach reduces the pilot overhead in channel estimation by extracting geometric channel parameters from visual sensing data.
Pioneering Future Wireless Technologies
Furthermore, SVWC enhances semantic signal compression by employing techniques such as image captioning to convert images into semantically dense feature vectors. This method significantly reduces transmission overhead by focusing on essential information, which is particularly useful for human-centric services like wireless brain-computer interfaces and intelligent humanoid robots. In the context of random access, SVWC utilizes crowd estimation to allocate a larger number of preambles to dense areas, thereby reducing collisions and access latency. This proactive approach ensures that the random access process remains efficient even in highly congested environments.
Simulation Results Showcase Efficacy
Simulation results presented in the paper demonstrate the effectiveness of SVWC in various scenarios. In beam management, SVWC achieves over a 91% reduction in positioning error and significant improvements in array gain compared to conventional 5G systems. For random access, SVWC reduces access latency by up to 29% in dense mobile environments. The data rate performance of SVWC also shows marked improvement, with an 84% increase over 5G NR cell association schemes, particularly in environments with high obstacle density.
Looking ahead, the paper identifies several future research directions for SVWC. One key challenge is ensuring seamless coverage even in demanding scenarios with obstacles, blind spots, or low-light conditions. Multi-modal sensing, which uses multiple sensing modalities simultaneously, can address this issue by enhancing detection accuracy. Another challenge is training SVWC models to be compatible with various wireless environments, which can be achieved using transfer learning to adapt pre-trained models with minimal data from specific environments. Privacy preservation is also crucial, as SVWC relies on visual sensing information. Approaches such as low-resolution sensing and privacy-preserving object detection can help mitigate privacy concerns. Finally, reducing the energy consumption and processing latency of SVWC is essential. Advances in AI processors and streamlined DL models like real-time DETR are expected to lower power consumption and latency, making SVWC more efficient.
The integration of sensing and computer vision in 6G wireless communications holds immense potential to enhance performance, reliability, and efficiency. As these technologies continue to evolve, SVWC is poised to become a cornerstone of future wireless communication systems, enabling faster, more accurate, and context-aware wireless services.
Visit Our Website: computer-vision-conferences.scifat.com
Twitter: twitter.com/Shulagna_sarkar
Pinterest: in.pinterest.com/computerconference22
Instagram: www.instagram.com/saisha.leo
Tumbler: www.tumblr.com/blog/shulagnasarkar22
YouTube: https://www.youtube.com/channel/UCUytaCzHX00QdGbrFvHv8zA
#computervision #deeplearning #machinelearning #artificialintelligence #neuralnetworks, #imageprocessing #objectdetection #imagerecognition #faceRecognition #augmentedreality #robotics #techtrends #3Dvision #professor #doctor #institute #sciencefather #researchawards #machinevision #visiontechnology #smartvision #patternrecognition #imageanalysis #semanticsegmentation #visualcomputing #datascience #techinnovation #university
No comments:
Post a Comment